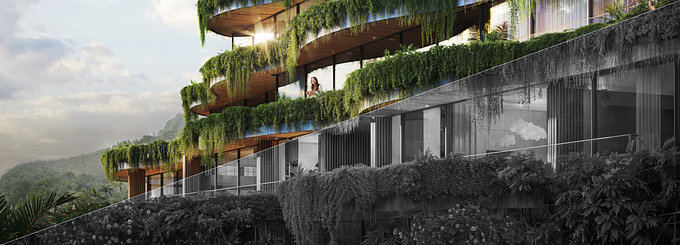
Articles
AI-Generated People in Renders: A New Era of Visualization
Traditional Methods of Adding People to Renders
Previously, two main methods were used to integrate people into visualizations: 3D models and 2D cutout images. In the first case, a visualizer added 3D models of people in 3ds Max, while in the second, they used images of real people sourced from specialized stock websites such as Vishopper and Cutout. Further processing of these images was done in Photoshop.
Adding 2D figures was often an unpopular task among visualizers. In addition to ensuring proper perspective, scale, and lighting, they had to meet client requirements regarding appearance, clothing, posture, and interactions between people in the scene. The main disadvantage of this approach was the difficulty of making modifications—changing a person’s smile or clothing was not possible, requiring a search for a new suitable figure instead. 3D models also had their downsides: they often appeared unnatural and "cardboard-like."
Adding 2D figures was often an unpopular task among visualizers. In addition to ensuring proper perspective, scale, and lighting, they had to meet client requirements regarding appearance, clothing, posture, and interactions between people in the scene. The main disadvantage of this approach was the difficulty of making modifications—changing a person’s smile or clothing was not possible, requiring a search for a new suitable figure instead. 3D models also had their downsides: they often appeared unnatural and "cardboard-like."
Advantages of Neural Networks
With the advent and development of neural networks, the process of adding people to renders has become significantly easier. Now, any modifications to a person's appearance can be easily made to meet the client's requests. For example, specific accessories, branded clothing, or facial expressions can be adjusted. Using neural networks, it is possible to:
- Create people from scratch;
- Generate realistic characters based on 3D models;
- Modify stock cutout images in any way necessary.


One of the key advantages of neural networks is their high-quality integration of people into renders. Simply sketching out light and shadow is enough for the neural network to generate the required character while considering these parameters.
In our work, we use Stable Diffusion locally on our devices, applying multiple nodes for fine-tuning the results. Occasionally, we also use upscale services and purchase prompts on specialized platforms for higher-quality generation.
The Process of Creating AI-Generated People
Creating realistic AI-generated people involves several steps:
- Defining client requirements. Determining the necessary characteristics such as age, appearance, clothing, mood, status, and scene narrative.
- Planning. The art director outlines the placement of people in the frame, their poses, and the overall storyline.
- Scene preparation. The visualizer inserts 3D people into the render or adds 2D figures. This stage has become much easier since the appearance and clothing of these figures are no longer important—everything can be adjusted using AI. The main focus is setting the right pose as the "foundation" for the future AI-generated person. In some cases, this step is skipped, and people are created entirely by AI.
- Generation and upscaling. Creating and refining characters to enhance their quality.
- Final adjustments. Modifying poses, placement, facial expressions, clothing, and other details.
Challenges and Limitations of AI-Generated People
Despite significant advantages, working with neural networks presents certain challenges:
- Difficulties with limbs. Even with advanced models like FLUX, generating realistic hands and feet can be problematic.
- Complex poses. Neural networks sometimes struggle to depict people in intricate poses, such as sitting on detailed or ornate furniture.
- Unpredictability. Even when following a proven generation and processing algorithm, neural networks can suddenly behave differently and produce incorrect results.
Conclusion
Thanks to neural networks, people in renders have become more diverse, modern, and customizable. Now, client preferences can be met with precision, down to specific clothing brands and accessories. In one project, for example, AI-generated people were customized to feature specific branded bracelets at the client’s request. The use of AI-generated people has also significantly reduced the number of revisions related to appearance, clothing, and poses, accelerating the visualization process and improving the overall quality of final images.
You must be logged in to post a comment. Login here.
About this article
AI-Generalist & Vis-oN studio / Mail: hello@vis-on.studio
visibility418
favorite_border0
mode_comment0